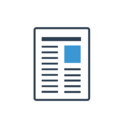
Annotation-Inspired Implicit Discourse Relation Classification with Auxiliary Discourse Connective Generation


Implicit discourse relation classification is a challenging task due to the absence of discourse connectives. To overcome this issue, we design an end-to-end neural model to explicitly generate discourse connectives for the task, inspired by the annotation process of PDTB. Specifically, our model jointly learns to generate discourse connectives between arguments and predict discourse relations based on the arguments and the generated connectives. To prevent our relation classifier from being misled by poor connectives generated at the early stage of training while alleviating the discrepancy between training and inference, we adopt Scheduled Sampling to the joint learning. We evaluate our method on three benchmarks, PDTB 2.0, PDTB 3.0, and PCC. Results show that our joint model significantly outperforms various baselines on three datasets, demonstrating its superiority for the task.
SEEK ID: https://publications.h-its.org/publications/1680
Research Groups: Natural Language Processing
Publication type: InProceedings
Citation: Proceedings of the 61st Annual Meeting of the Association for Computational Linguistics, Toronto, Ontario, Canada, July 2023, pp. 15696-15712
Date Published: 8th Jul 2023
URL: https://aclanthology.org/2023.acl-long.874.pdf
Registered Mode: manually

Views: 2380
Created: 31st May 2023 at 11:38
Last updated: 5th Mar 2024 at 21:25

This item has not yet been tagged.

None