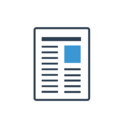
High-Performance approaches for Phylogenetic Placement, and its application to species and diversity quantification

In recent years, advances in high-throughput genetic sequencing, coupled with the ongoing exponential growth and availability of computational resources, have enabled entirely new approaches in the biological sciences. It is now possible to perform broad sequencing of the genetic content of entire communities of organisms from individual environmental samples. Such methods are particularly relevant to microbiology. The field was previously largely constrained to the study of those microbes that could be cultured in the laboratory (i.e., in vitro), which represents a small fraction of the diversity observed in nature.
In contrast to this, high-throughput sequencing now enables the collection of genetic sequences directly from a microbiome in its natural environment (i.e., in situ).
A typical goal of microbiome studies is the taxonomic classification of the sequences contained in a sample (the queries). Phylogenetic methods are commonly used to determine detailed taxonomic relationships between queries and well-trusted reference sequences from previously classified organisms. However, due to the high volume (106 to 109) of query sequences produced by high-throughput sequencing based microbiome sampling, accurate phylogenetic tree reconstruction is computationally infeasible. Moreover, currently used sequencing technologies typically produce short query sequences that have limited phylogenetic signal, causing instability in the inference of comprehensive phylogenies.
Another common goal of microbiome studies is to quantify the diversity within a sample, as well as between multiple samples. Phylogenetic methods are commonly used for this task as well, typically involving the inference of a phylogenetic tree comprising all query sequences, or a clustered subset thereof. Again, as with taxonomic identification, analyses based on this kind of tree inference may result in inaccurate results, and/or be computationally prohibitive.
In contrast to comprehensive phylogenetic inference, phylogenetic placement is a method that identifies the phylogenetic context of a query sequence within a trusted reference tree. Such methods typically regard the reference tree as immutable, that is the reference tree is not altered before, during, or after the placement of a query. This allows for the phylogenetic placement of a query sequence in linear time with respect to the size of the reference tree. When combined with taxonomic information for the reference sequences, phylogenetic placement therefore allows to identify a query. Further, phylogenetic placement enables a wealth of additional post-analysis procedures, allowing for example the association of microbiome characteristics with clinical diagnostic properties.
In this thesis I present my work on designing, implementing, and improving EPA-ng, a high-performance implementation of maximum likelihood phylogenetic placement. EPA-ng is designed to scale to billions of input query sequences and to parallelize across thousands of cores in both shared, and distributed memory environments. It also improves the single-core processing speed by up to 30 times compared to its closest direct competitors. Recently, we have introduced an optional feature to EPA-ng that allows for placement into substantially larger reference trees, using an active memory management approach that trades memory for execution time.
Additionally, I present a massively parallel approach to quantify the diversity of a sample, based on phylogenetic placement results. the resulting tool, called SCRAPP, combines state-of-the-art methods for maximum likelihood phylogenetic tree inference and molecular species delimitation to infer a species count distribution on a reference tree for a given sample. Furthermore, it employs a novel approach for clustering placement results, allowing the user to reduce the computational effort.
SEEK ID: https://publications.h-its.org/publications/1434
Research Groups: Computational Molecular Evolution
Publication type: Doctoral Thesis
Citation:
Date Published: No date defined
URL: https://publikationen.bibliothek.kit.edu/1000139668
Registered Mode: manually

Views: 5330
Created: 18th Jan 2022 at 08:51
Last updated: 5th Mar 2024 at 21:24

This item has not yet been tagged.

None