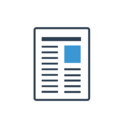
Much Ado About Nothing: Accelerating Maximum Likelihood Phylogenetic Inference via Early Stopping to evade (Over-)optimization


Abstract:
Maximum Likelihood (ML) based phylogenetic inference constitutes a challenging optimization problem. Given a set of aligned input sequences, phylogenetic inference tools strive to determine the tree topology, the branch-lengths, and the evolutionary parameters that maximize the phylogenetic likelihood function. However, there exist compelling reasons to not push optimization to its limits, by means of early, yet adequate stopping criteria. Since input sequences are typically subject to stochastic and systematic noise, one should exhibit caution regarding (over-)optimization and the inherent risk of overfitting the model to noisy input data. To this end, we propose, implement, and evaluate four statistical early stopping criteria in RAxML-NG that evade excessive and compute-intensive (over-)optimization. These generic criteria can seamlessly be integrated into other phylo-genetic inference tools while not decreasing tree accuracy. The first two criteria quantify input data-specific sampling noise to derive a stopping threshold. The third, employs the Kishino-Hasegawa (KH) test to statistically assess the significance of differences between intermediate trees
before
, and
after
major optimization steps in RAxML-NG. The optimization terminates early when improvements are insignificant. The fourth method utilizes multiple testing correction in the KH test. We show that all early stopping criteria infer trees that are statistically equivalent compared to inferences without early stopping. In conjunction with a necessary simplification of the standard RAxML-NG tree search heuristic, the average inference times on empirical and simulated datasets are ∼3.5 and ∼1.8 times faster, respectively, than for standard RAxML-NG v.1.2. The four stopping criteria have been implemented in RAxML-NG and are available as open source code under GNU GPL at
https://github.com/togkousa/raxml-ng
.
SEEK ID: https://publications.h-its.org/publications/1879
DOI: 10.1101/2024.07.04.602058
Research Groups: Computational Molecular Evolution
Publication type: Journal
Citation: biorxiv;2024.07.04.602058v1,[Preprint]
Date Published: 8th Jul 2024
Registered Mode: by DOI

Citation
Togkousidis, A., Stamatakis, A., & Gascuel, O. (2024). Much Ado About Nothing: Accelerating Maximum Likelihood Phylogenetic Inference via Early Stopping to evade (Over-)optimization. In []. Cold Spring Harbor Laboratory. https://doi.org/10.1101/2024.07.04.602058
Activity
Views: 978
Created: 16th Oct 2024 at 13:28
Last updated: 16th Oct 2024 at 13:28

This item has not yet been tagged.

None