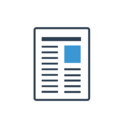
KineticNet: Deep learning a transferable kinetic energy functional for orbital-free density functional theory


Abstract:
Orbital-free density functional theory (OF-DFT) holds promise to compute ground state molecular properties at minimal cost. However, it has been held back by our inability to compute the kinetic energy as a functional of electron density alone. Here, we set out to learn the kinetic energy functional from ground truth provided by the more expensive Kohn–Sham density functional theory. Such learning is confronted with two key challenges: Giving the model sufficient expressivity and spatial context while limiting the memory footprint to afford computations on a GPU and creating a sufficiently broad distribution of training data to enable iterative density optimization even when starting from a poor initial guess. In response, we introduce KineticNet, an equivariant deep neural network architecture based on point convolutions adapted to the prediction of quantities on molecular quadrature grids. Important contributions include convolution filters with sufficient spatial resolution in the vicinity of nuclear cusp, an atom-centric sparse but expressive architecture that relays information across multiple bond lengths, and a new strategy to generate varied training data by finding ground state densities in the face of perturbations by a random external potential. KineticNet achieves, for the first time, chemical accuracy of the learned functionals across input densities and geometries of tiny molecules. For two-electron systems, we additionally demonstrate OF-DFT density optimization with chemical accuracy.
SEEK ID: https://publications.h-its.org/publications/1822
DOI: 10.1063/5.0158275
Research Groups: SIMPLAIX
Publication type: Journal
Journal: The Journal of Chemical Physics
Citation: The Journal of Chemical Physics 159(14),144113
Date Published: 14th Oct 2023
Registered Mode: by DOI

Citation
Remme, R., Kaczun, T., Scheurer, M., Dreuw, A., & Hamprecht, F. A. (2023). KineticNet: Deep learning a transferable kinetic energy functional for orbital-free density functional theory. In The Journal of Chemical Physics (Vol. 159, Issue 14). AIP Publishing. https://doi.org/10.1063/5.0158275
Activity
Views: 955
Created: 13th Mar 2024 at 10:05
Last updated: 13th Mar 2024 at 10:06

This item has not yet been tagged.

None