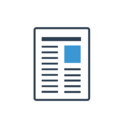
Scalable stellar evolution forecasting - Deep learning emulation versus hierarchical nearest-neighbor interpolation


Many astrophysical applications require efficient yet reliable forecasts of stellar evolution tracks. One example is population synthesis, which generates forward predictions of models for comparison with observations. The majority of state-of-the-art rapid population synthesis methods are based on analytic fitting formulae to stellar evolution tracks that are computationally cheap to sample statistically over a continuous parameter range. The computational costs of running detailed stellar evolution codes, such as MESA, over wide and densely sampled parameter grids are prohibitive, while stellar-age based interpolation in-between sparsely sampled grid points leads to intolerably large systematic prediction errors. In this work, we provide two solutions for automated interpolation methods that offer satisfactory trade-off points between cost-efficiency and accuracy. We construct a timescale-adapted evolutionary coordinate and use it in a two-step interpolation scheme that traces the evolution of stars from zero age main sequence all the way to the end of core helium burning while covering a mass range from 0.65 to 300 M⊙. The feedforward neural network regression model (first solution) that we train to predict stellar surface variables can make millions of predictions, sufficiently accurate over the entire parameter space, within tens of seconds on a 4-core CPU. The hierarchical nearest-neighbor interpolation algorithm (second solution) that we hard-code to the same end achieves even higher predictive accuracy, the same algorithm remains applicable to all stellar variables evolved over time, but it is two orders of magnitude slower. Our methodological framework is demonstrated to work on the MESA Isochrones and Stellar Tracks (Choi et al. 2016) data set, but is independent of the input stellar catalog. Finally, we discuss the prospective applications of these methods and provide guidelines for generalizing them to higher dimensional parameter spaces.
SEEK ID: https://publications.h-its.org/publications/1794
DOI: 10.1051/0004-6361/202347118
Research Groups: Physics of Stellar Objects
Publication type: Journal
Journal: A&A
Citation: A&A 681:A86
Date Published: 2024
URL: https://doi.org/10.1051/0004-6361/202347118
Registered Mode: imported from a bibtex file

Views: 3207
Created: 15th Feb 2024 at 15:35
Last updated: 5th Mar 2024 at 21:25

This item has not yet been tagged.

None