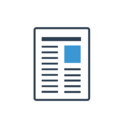
Asteroid: a new algorithm to infer species trees from gene trees under high proportions of missing data


Abstract
Motivation
Missing data and incomplete lineage sorting (ILS) are two major obstacles to accurate species tree inference. Gene tree summary methods such as ASTRAL and ASTRID have been developed to account for ILS. However, they can be severely affected by high levels of missing data.
Results
We present Asteroid, a novel algorithm that infers an unrooted species tree from a set of unrooted gene trees. We show on both empirical and simulated datasets that Asteroid is substantially more accurate than ASTRAL and ASTRID for very high proportions (>80%) of missing data. Asteroid is several orders of magnitude faster than ASTRAL for datasets that contain thousands of genes. It offers advanced features such as parallelization, support value computation and support for multi-copy and multifurcating gene trees.
Availability and implementation
Asteroid is freely available at https://github.com/BenoitMorel/Asteroid.
Supplementary information
Supplementary data are available at Bioinformatics online.
SEEK ID: https://publications.h-its.org/publications/1744
DOI: 10.1093/bioinformatics/btac832
Research Groups: Computational Molecular Evolution
Publication type: Journal
Journal: Bioinformatics
Editors: Russell Schwartz
Citation: Bioinformatics 39(1),btac832
Date Published: 2023
Registered Mode: by DOI

Views: 474
Created: 2nd Jan 2024 at 18:21
Last updated: 5th Mar 2024 at 21:25

This item has not yet been tagged.

None