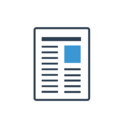
High-Throughput Phenotypic Screening and Machine Learning Methods Enabled the Selection of Broad-Spectrum Low-Toxicity Antitrypanosomatidic Agents.


Broad-spectrum anti-infective chemotherapy agents with activity against Trypanosomes, Leishmania, and Mycobacterium tuberculosis species were identified from a high-throughput phenotypic screening program of the 456 compounds belonging to the Ty-Box, an in-house industry database. Compound characterization using machine learning approaches enabled the identification and synthesis of 44 compounds with broad-spectrum antiparasitic activity and minimal toxicity against Trypanosoma brucei, Leishmania Infantum, and Trypanosoma cruzi. In vitro studies confirmed the predictive models identified in compound 40 which emerged as a new lead, featured by an innovative N-(5-pyrimidinyl)benzenesulfonamide scaffold and promising low micromolar activity against two parasites and low toxicity. Given the volume and complexity of data generated by the diverse high-throughput screening assays performed on the compounds of the Ty-Box library, the chemoinformatic and machine learning tools enabled the selection of compounds eligible for further evaluation of their biological and toxicological activities and aided in the decision-making process toward the design and optimization of the identified lead.
SEEK ID: https://publications.h-its.org/publications/1734
PubMed ID: 37921561
Research Groups: Scientific Databases and Visualisation
Publication type: Journal
Journal: J Med Chem
Citation: J Med Chem. 2023 Nov 3. doi: 10.1021/acs.jmedchem.3c01322.
Date Published: 3rd Nov 2023
Registered Mode: by PubMed ID

Views: 1222
Created: 6th Nov 2023 at 10:06
Last updated: 5th Mar 2024 at 21:25

This item has not yet been tagged.

None