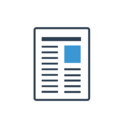
Unlocking the Potential: Predicting Redox Behavior of Organic Molecules, from Linear Fits to Neural Networks


Redox-active organic molecules, i.e., molecules that can relatively easily accept and/or donate electrons, are ubiquitous in biology, chemical synthesis, and electronic and spintronic devices, such as solar cells and rechargeable batteries, etc. Choosing the best candidates from an essentially infinite chemical space for experimental testing in a target application requires efficient screening approaches. In this Review, we discuss modern in silico techniques for predicting reduction and oxidation potentials of organic molecules that go beyond conventional first-principles computations and thermodynamic cycles. Approaches ranging from simple linear fits based on molecular orbital energy approximation and energy difference approximation to advanced regression and neural network machine learning algorithms employing complex descriptors of molecular compositions, geometries, and electronic structures are examined in conjunction with relevant literature examples. We discuss the interplay between ab initio data and machine learning (ML), i.e., whether it is better to base predictions on low-level quantum-chemical results corrected with ML or to bypass first-principles computations entirely and instead rely on elaborate deep learning architectures. Finally, we list currently available data sets of redox-active organic molecules and their experimental and/or computed properties to facilitate the development of screening platforms and rational design of redox-active organic molecules.
SEEK ID: https://publications.h-its.org/publications/1685
Filename: 2023_Fedorov_JCTC.pdf
Format: PDF document
Size: 12.9 MB
SEEK ID: https://publications.h-its.org/publications/1685
Research Groups: Computational Carbon Chemistry, SIMPLAIX
Publication type: Journal
Journal: Journal of Chemical Theory and Computation
Citation: J. Chem. Theory Comput. 19(15):4796-4814
Date Published: 8th Aug 2023
Registered Mode: by DOI

Views: 2849 Downloads: 1
Created: 4th Aug 2023 at 09:53
Last updated: 11th Mar 2024 at 13:33

This item has not yet been tagged.

None