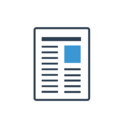
Modeling Structural Similarities between Documents for Coherence Assessment with Graph Convolutional Networks


Coherence is an important aspect of text quality, and various approaches have been applied to coherence modeling. However, existing methods solely focus on a single document’s coherence patterns, ignoring the underlying correlation between documents. We investigate a GCN-based coherence model that is capable of capturing structural similarities between documents. Our model first identifies the graph structure of each document, from where we mine different sub-graph patterns. We then construct a heterogeneous graph for the training corpus, connecting documents based on their shared subgraphs. Finally, a GCN is applied to the heterogeneous graph to model the connectivity relationships. We evaluate our method on two tasks, assessing discourse coherence and automated essay scoring. Results show that our GCN-based model outperforms baselines, achieving a new state-of-the-art on both tasks.
SEEK ID: https://publications.h-its.org/publications/1679
Research Groups: Natural Language Processing
Publication type: InProceedings
Citation: Proceedings of the 61st Annual Meeting of the Association for Computational Linguistics, Toronto, Ontario, Canada, July 2023, pp. 7792-7808
Date Published: 8th Jul 2023
URL: https://aclanthology.org/2023.acl-long.431.pdf
Registered Mode: manually

Views: 3839
Created: 31st May 2023 at 11:36
Last updated: 5th Mar 2024 at 21:25

This item has not yet been tagged.

None