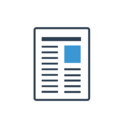
White Paper: Towards in silico approaches for personalised medicine – Recommendations for verifying and validating predictive computational models in EU collaborative research


Harmonization of data integration is the key to standardization efforts in personalised medicine, which would also facilitate cross-European studies. Standardization of the models themselves is less essential within a research context, where new models are created and tested in line with research progress, harmonization and/or standardization of input data is both feasible and necessary.
We argue that model validation should receive more attention, and other measures should be implemented such that validation of models within personalised medicine becomes easier, also across borders. While this is an evident necessity within the context of models implemented as medical devices or decision tools, which are regulated by the European Medicines Agency and national competent authorities, we argue that model validation should be a higher priority at research level also, facilitating assessment by peers and by medical doctors – who themselves should receive better training in assessment of research using in silico models. This will also ease the implementation of translational research results in the clinic.
Acceptance by doctors and the relevant medical specialties is a key hurdle for in silico models in personalised medicine. Any medical product - device, algorithm or drug - has to prove itself safe and effective to be licensed for use by regulators; however, it has also to be accepted by medical experts as being a good choice, and be recommended within clinical specialties.
EU-STANDS4PM joined forces to examine to what extent existing standards or standards under development for both format and semantics can be used to link clinical and health as well as research data to computational models relevant for personalised medicine. As all requirements should be equally understood and fulfilled by users it is important to define them uniformly in an international context. To achieve this the conclusion of our work shall be also discussed in international standardization and technical committees, especially in the case of standards that are still being drawn up, and new standardization projects shall be initiated where necessary.
We present a White Paper featuring recommendations for standardization of data integration as well as recommendations for standardization of model validation within a collaborative research context, such that health-related data can be optimally used for translational research and personalised medicine across Europe.
As such the White Paper showcases the approach that takes big data in health through harmonized data integration to the most relevant predictive computational models for personalised medicine. As they are refined and validated these models can provide guidance not just how to use data, but also how to best cope with disease and preserve wellbeing in the daily lives of patients.
SEEK ID: https://publications.h-its.org/publications/1667
Research Groups: Scientific Databases and Visualisation
Publication type: Misc
Publisher: Zenodo
Citation:
Date Published: 7th Feb 2022
URL: https://doi.org/10.5281/zenodo.5995853
Registered Mode: manually

Views: 4427
Created: 24th May 2023 at 11:10
Last updated: 5th Mar 2024 at 21:25

None