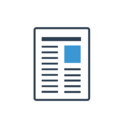
Review of Deep Learning Methods for Individual Treatment Effect Estimation with Automatic Hyperparameter Optimization


Abstract:
<p>Abstract—Estimation of individual treatment effect (ITE) for different types of treatment is a common challenge in therapy assessments, clinical trials and diagnosis. Deep learning methods, namely representation based, adversarial, and variational, have shown promising potential in ITE estimation. However, it was unclear whether the hyperparameters of the originally proposed methods were well optimized for different benchmark datasets. To solve these problems, we created a public code library containing representation-based, adversarial, and variational methods written in TensorFlow. In order to have a broader collection of ITE estimation methods, we have also included neural network based meta-learners. The code library is made accessible for reproducibility and facilitating future works in the field of causal inference. Our results demonstrate that performance of most methods can be improved using automatic hyperparameter optimization. Additionally, we review the methods and compare the performance of the optimized models from our library on publicly available datasets. The potential of hyperparameter optimization may encourage researchers to focus on this aspect when creating new methods for inferring individual treatment effect.</p>
SEEK ID: https://publications.h-its.org/publications/1615
DOI: 10.36227/techrxiv.20448768.v2
Research Groups: Data Mining and Uncertainty Quantification
Publication type: Misc
Citation: [Preprint]
Date Published: 6th Dec 2022
Registered Mode: by DOI

Citation
Sirazitdinov, A., Buchwald, M., Hesser, J., & Heuveline, V. (2022). Review of Deep Learning Methods for Individual Treatment Effect Estimation with Automatic Hyperparameter Optimization. In []. Institute of Electrical and Electronics Engineers (IEEE). https://doi.org/10.36227/techrxiv.20448768.v2
Activity
Views: 4038
Created: 15th Feb 2023 at 14:13
Last updated: 5th Mar 2024 at 21:25

This item has not yet been tagged.

None