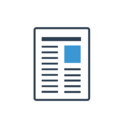
RASPD+: Fast Protein-Ligand Binding Free Energy Prediction Using Simplified Physicochemical Features


The virtual screening of large numbers of compounds against target protein binding sites has become an integral component of drug discovery workflows. This screening is often done by computationally docking ligands into a protein binding site of interest, but this has the drawback of a large number of poses that must be evaluated to obtain accurate estimates of protein-ligand binding affinity. We here introduce a fast pre-filtering method for ligand prioritization that is based on a set of machine learning models and uses simple pose-invariant physicochemical descriptors of the ligands and the protein binding pocket. Our method, Rapid Screening with Physicochemical Descriptors + machine learning (RASPD+), is trained on PDBbind data and achieves a regression performance that is better than that of the original RASPD method and traditional scoring functions on a range of different test sets without the need for generating ligand poses. Additionally, we use RASPD+ to identify molecular features important for binding affinity and assess the ability of RASPD+ to enrich active molecules from decoys.
SEEK ID: https://publications.h-its.org/publications/1159
DOI: 10.3389/fmolb.2020.601065
Research Groups: Molecular and Cellular Modeling
Publication type: Journal
Journal: Frontiers in Molecular Biosciences
Citation: Frontiers in Molecular Biosciences 7:393
Date Published: 2020
URL: https://www.frontiersin.org/article/10.3389/fmolb.2020.601065
Registered Mode: imported from a bibtex file

Views: 4572
Created: 17th Dec 2020 at 09:53
Last updated: 5th Mar 2024 at 21:24

This item has not yet been tagged.

None